In the maze of modern healthcare research, reproducibility remains a crucial marker of sound science. Yet, it’s a marker we often miss. Medical breakthroughs built on shaky, producible findings could have dire consequences, from a false sense of progress to misguiding clinical practices. Enter AI, the beacon of integrity and reliability in healthcare research.Â
With its statistical modeling, deep learning, and data analysis prowess, AI stands at the forefront of solving the reproducibility puzzle and redefining how we approach medical science. Partnering with the best AI consulting firms can provide invaluable expertise and guidance when addressing these challenges.
The Reproducibility Labyrinth in Healthcare Research
The reproducibility crisis is a pervasive challenge that has significantly impacted trust in scientific results. The crisis has catalyzed a reflective moment in healthcare, a field where precision is everything. Research findings, including those related to digital health technologies, often come under the microscope—sometimes literally—and what’s discovered isn’t always comforting.
From the variability and methodological issues in in-vivo studies to the complex interplay of individual patient factors in clinical trials, the road to reproducibility is fraught with pitfalls. These challenges create an environment where one cannot be certain that a study’s findings can be repeated with comparable results, raising doubts about the reliability of our advances.
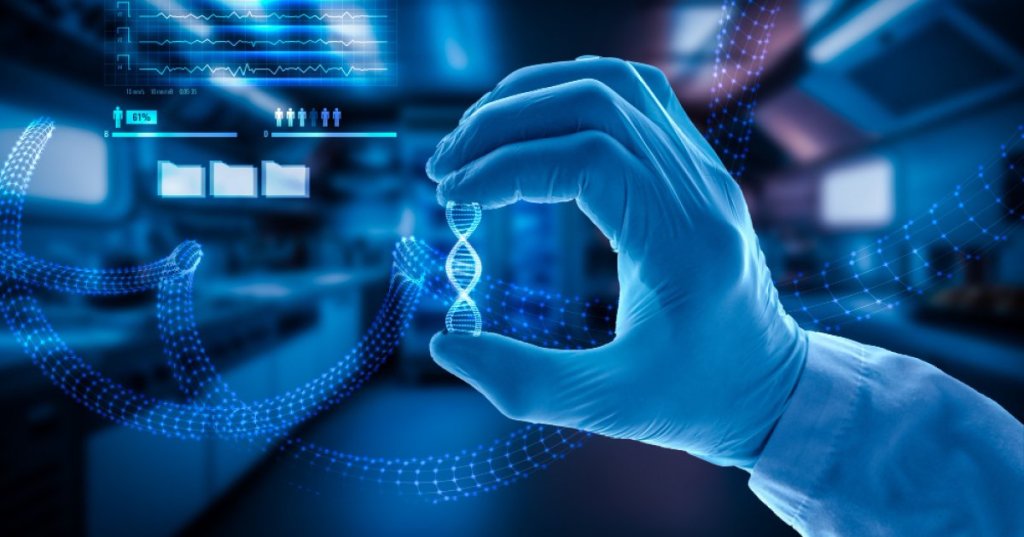
The healthcare crisis isn’t just a scientific hiccup; it’s a stumbling block that impedes the pace of innovation and endangers patient care. It necessitates a transformation in methodology and tools that redefine rigor in medical research.
A Beacon for Reproducibility
AI companies in Pakistan are more than just a buzzword—they’re the intelligent designer of a new research paradigm. It leverages machine learning algorithms capable of detecting trends and patterns in data beyond human perception. In doing so, AI reinforces the foundational pillars of scientific research with unprecedented levels of algorithmic reproducibility.
Defining AI’s Role in Research Integrity
While AI is not immune to the ‘garbage in, garbage out’ dilemma, its potential to ingest and interpret data at an industrial scale is unparalleled. Organized datasets, free from human error yet curated by human oversight, provide fertile ground for AI to perform analyses with consistent precision.
AI can transform the ambiguity of methodological diversity into a robust framework where reproducibility is not just possible but expected. It also can standardize complex processes, reducing the margin of error and bias inherent in manual experimentation.
AI in Data Analysis and Interpretation
One area where AI excels is in data analysis, particularly in ‘omics’ sciences such as genomics, proteomics, and metabolomics. The deluge of data points that would leave any human drowned in confusion is to AI but a vast sea of statistical possibility.
AI algorithms can sort, categorize, and identify correlations in data with an efficiency that humans can’t match. By automating these processes, AI ensures an impartial approach to analysis, free from subconscious human bias.
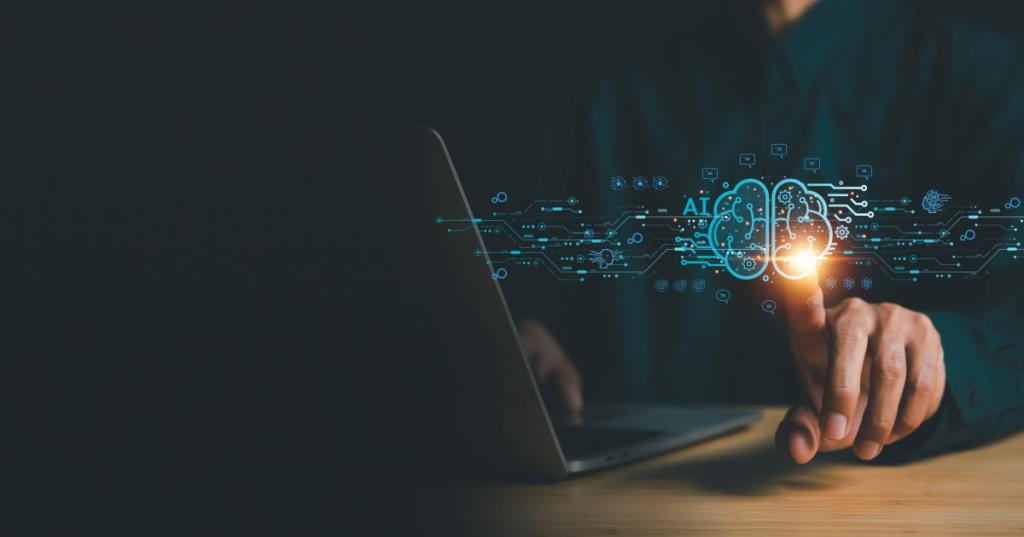
AI for Automated Experiment Replication
AI’s contribution to reproducibility extends to automating experiment processes. With the help of big data in healthcare, robotic arms guided by AI protocols can replicate experiments with precision down to the micrometer, ensuring that the minutest of variables are accounted for.
Furthermore, AI can monitor and analyze these replications without fatigue, reporting the slightest deviation in results that could tip the scales of scientific reproducibility.
Benefits of AI:
Integrating AI in healthcare research isn’t just about solving the reproducibility crisis; it brings many benefits that enhance the entire research lifecycle.
Precision and Reliability
By integrating AI into research methodologies, we can achieve levels of accuracy and reliability that traditional methods could only dream of. AI’s ability to control variables with meticulous precision and easily sift through vast datasets helps build studies on solid, reproducible foundations.

The Efficiency Factor
AI isn’t just a better method; it’s a faster one. Reducing the time between hypothesis and conclusion accelerates the pace of research, pushing ideas into the realms of reproducibility faster and, consequently, into the hands of those who need them—patients and practitioners.
Unveiling Unseen Patterns
Medical breakthroughs often hinge on discovering subtle, often overlooked patterns within data noise. AI’s pattern-detecting capabilities are a beacon, illuminating pathways to reproducible discoveries that might have remained concealed from human eyes.
Pharmaceuticals on the Fast Track
The path to drug discovery is laden with uncertainty. AI serves as a guide, identifying potential drug candidates with greater precision and at an accelerated pace, reducing the costs of trial and error associated with traditional, less predictive methods.
Challenges of AI Integration
Despite its promise, adopting AI in healthcare research is not without concerns, and navigating these challenges is crucial for its successful implementation.

Ethical Considerations and Algorithmic Bias
AI systems are only as good as the data they’re trained on, and if that data is biased, so too will the AI. Addressing ethical dilemmas and ensuring the neutrality of AI’s recommendations is a critical first step.
Data Quality and Accessibility
The cornerstone of AI is data, and healthcare has its share of data issues. From siloed systems to the lack of standardized data formats, the journey toward AI-reliant research is paved with challenges of data quality and accessibility.
Integrating AI with Legacy Systems
The healthcare industry is not a hotbed of rapid technological adaptation. Legacy systems in technology and mindset present formidable obstacles to AI’s seamless integration into existing research frameworks.
Winning Hearts and Minds
The most potent AI system is only as effective as those who use it. Overcoming skepticism and winning the trust of healthcare professionals is a necessary step in the AI revolution.
AI in Action
Numerous case studies demonstrating AI’s potential and feasibility in healthcare research show that AI has made undeniable impacts.
Genomic Research
AI has transformed the field of genomics, accelerating the identification of genetic markers and enhancing our understanding of complex conditions like cancer, opening new avenues for personalized treatments that were mere conjecture a decade ago.

Diagnostic Solutions
AI-driven diagnostic tools are entering the market, providing practitioners with reproducible, unbiased insights that aid in detecting and characterizing diseases, potentially saving lives with timelier interventions.
Predictive Analytics
By analyzing historical and real-time data, AI can provide reproducible predictions of disease outbreaks and patient outcomes, empowering health organizations to prepare and respond effectively.
Conclusion:
The AI revolution in healthcare research is not a question of if but when. As we stand at the cusp of an era where machines play an integral role in the reproducibility of our most critical findings, the imperative to embrace this change becomes more apparent.
With the power to redefine the rigor and reliability of our research, AI offers a clear path forward in an industry fraught with reproducibility challenges. By integrating AI into our healthcare research, we not only solve the current crisis but also lay the groundwork for a future of scientific and medical discovery that is as sound as it is profound.
It is up to us, the torchbearers of healthcare research, to wield this tool with wisdom and foresight. Let us embrace the transformative power of AI to not only address the reproducibility crisis, but also enhance patient care, improve public health, and enrich the human experience with the wealth of reproducibility derived medical knowledge.
Leave a comment